Automation, Artificial Intelligence and Machine Learning Should Also Apply to Fraud Detection and Removal
Warning: There are a lot of acronyms in this post that are used interchangeably in the mainstream. However, these terms—artificial intelligence (AI), analytics, machine learning (ML), and automation—are actually more symbiotic than they interchangeable. In the security world, these functions work together to detect, analyze and make judgements on cyberattacks, threats and vulnerabilities.
Organizations recognize the importance of these capabilities in their security programs. For example budgets for automation are up 3 to 10% over 2019 budgets, according to a recent SANS survey.
Yet, when it comes to using these capabilities for online fraud detection, most organizations are behind the fraudsters. It’s time for customer-facing organizations to utilize AI and ML to detect, analyze and learn from growing forms of fraud that affects their brands and customers (such as fake pharmacies, tech support scams, cryptojacking, counterfeiting, and of course, phishing).
Awakening
Anti-fraud groups are starting to understand the importance of AI and ML in their anti-fraud and brand protection programs. They’re just not spending there—yet. For example, 93% of respondents to a survey of 200 brands believe AI will have a positive and cost-reductive impact on their anti-fraud efforts. Another Anti-Fraud Technology report indicates that AI and ML are under-adopted, but AI/ML also represents the largest predicted growth area among respondents over the next two years.
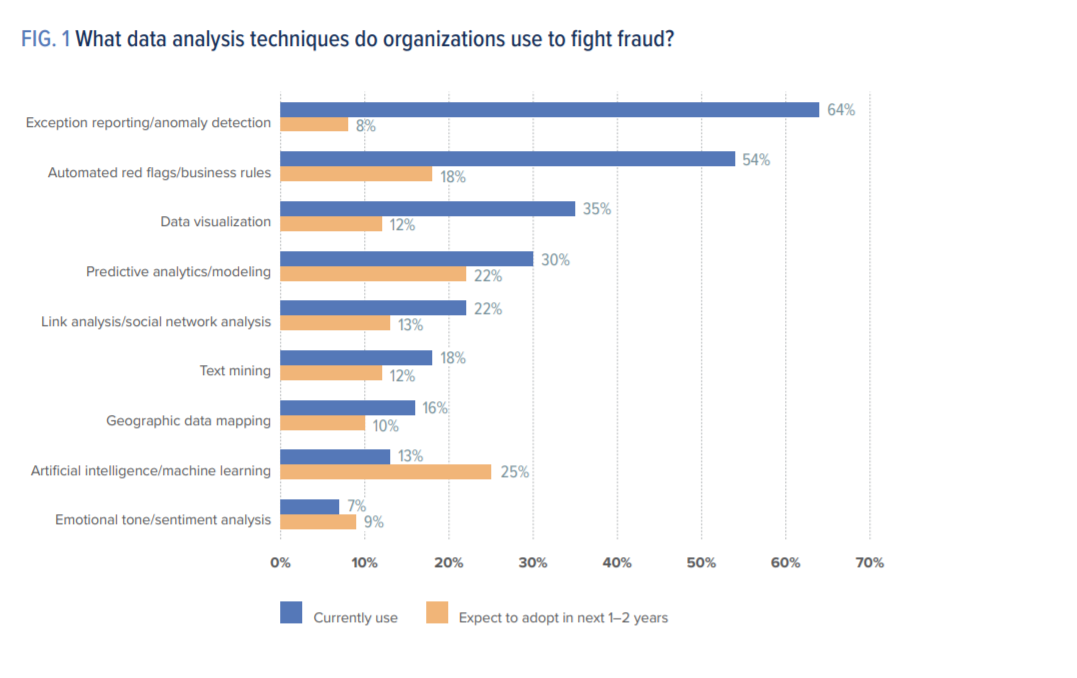
The same report highlights that automation, predictive analytics, red flag detection, link analysis, and online evidence capture are also commonly utilized to detect fraud. Respondents felt that automating these processes improves capacity, accuracy, efficiency and timeliness.
AI and Machine Learning Applied
When applied to detecting online fraud, AI and ML looks a little different than how these capabilities are used in traditional SOC support. For example, instead of looking for patterns and IOC’s, AI is trained on domains and URLS, content, images, and images of images (copies of websites). So, the key features of an AI engine used in anti-fraud would:
· Support fast scanning and analysis of sites on the web, in the cloud, and on social media.
· Analyze URLs, shortened URLs (bitly, etc.), email lures, even information left in a victim’s browser when visit their real place of business (which is useful intelligence).
· Thoroughly analyze sites for evidence of fraudulent intent and use.
· Analyze against top categories of fraud types as well as adapt to new categories of fraud.
· Detect and analyze squatter domains using a version of the brand name even before a site goes up.
Based on the analysis and data sets to support that analysis, ML then makes the determination of the site’s intent. It should be constantly learning and updating its own data sets of fraud traits, as well as updating blocklists that can be continually monitored and enforced against.
Continuous Loop
Just as ML learns through human-understandable indicators of fraud, it should also report output in human-understandable fashion. These reports and the related output should then integrate with threat hunting and other detection activities to help serve the SOC and fraud detection units for trending and analysis.
Right now, the fraudsters are steps ahead of the organizations they impersonate in order to commit fraud. The key to protection is quick detection, analysis and determination of a site’s intent—all of which Bolster has enabled through automation, AI, and machine learning.
You can read more about how our AI works here.